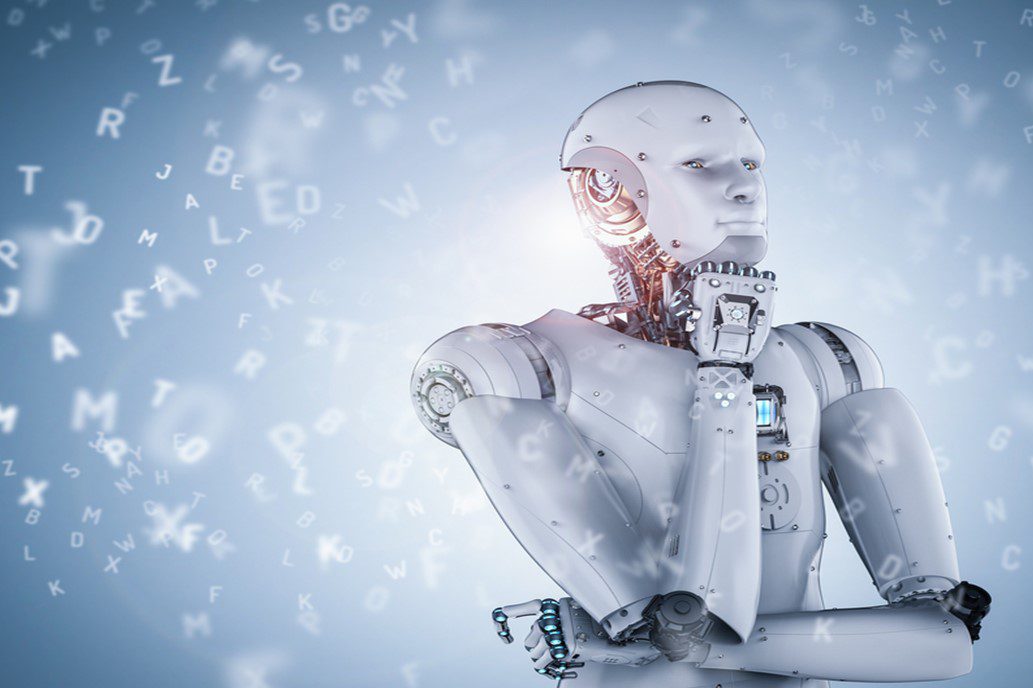
Should professional translators shun machine translation?
by Steve Dept, cApStAn CEO
Beyond the AI hype and controversial reports about automatic translation achieving parity with human translation, neural machine translation (NMT) has undoubtedly made spectacular progress in the last three years. Should professional translators resist or should they use it?
Machine translation software has been around for a long time. In the field of natural language processing (NLP), researchers investigated how to program computers to analyze and understand human language. Rule-based machine translation appears in 1954: the Georgetown-IBM experiment translated 60 sentences from Russian to English. Claims were made at the time that machine translation would be a solved problem within half a decade. Rule-based machine translation was rather successful: Systran was the first commercial machine translation system, and the European Commission developed a Systran-based system for English to French as of 1976. I personally post-edited thousands of pages of Systran machine output. Rather frustrating, I recall.
Statistical machine translation (SMT) made its appearance when more text became available in several languages and in electronic form, and as processors became faster: millions of sentences and their likely translation could be analyzed quickly, so that the translation machine would “predict” a plausible translation output of input in the form of monolingual text. I recall that, in 2000, knowledgeable people claimed that by 2015 there would be no human translators anymore. In 2015, computer scientists started saying that human translators were unlikely to disappear. Then, in 2017, came the advent of neural machine translation (NMT), which uses deep learning, i.e. machine learning with hidden layers, to create its own path towards solving a translation problem.
As shown below, “machine learning” (ML) is a subset of artificial intelligence (AI). In translation, ML may be used to identify recurring patterns in the human translator’s choices, which helps the system predict a higher likelihood for one possible translation versus another. Deep learning is a subset of machine learning: the programmers cannot predict how the computer will use the ML algorithms.
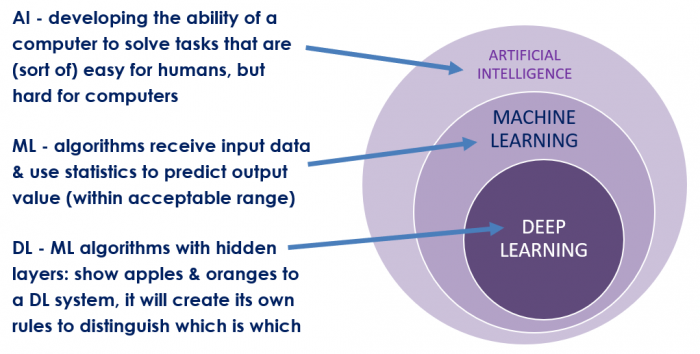
In the field of machine translation, the advances are real. The opportunities are “more, faster”. One of the threats with neural machine translation is “a new level of fluency that does its best to hide mistranslations”. One needs to remember that the translation engine does not understand causality and cannot infer from the context. Therefore, disambiguation of the source text is key. Without help, the translation machine cannot decide whether a <Russian teacher> actually teaches Russian or is a Russian person who might teach any subject.
At this stage, there are perhaps too many articles that benchmark one machine translation system against another or pitch machine translation against human translation, and there too little testing of those models of man-machine interaction that do produce high-quality translations with shorter turnaround times. So, what is my answer to the question “should professional translators shun machine translation?” Don’t shun it, embrace it, use it! When there is little leverage from translation memories, the contribution of neural machine translation can be really high. Today, many NMT engines can be called up from most computer-assisted translation tools (CAT tools). Using machine translation with discernment can boost a translator’s productivity without compromising in quality.
Translation work will evolve, it is already changing fast. Linguists will have new and more exciting tasks, and a higher level of specialization will be required. At cApStAn we already help organizations disambiguate source material to make it more translatable. We help prepare contextual elements for both human translators and machine translation engines to interpret. We set up automated quality assurance checks that increase consistency and perform repetitive tasks such as harmonizing quotation marks, checking whether all segments are translated, or checking adherence to a glossary. Exciting times ahead!