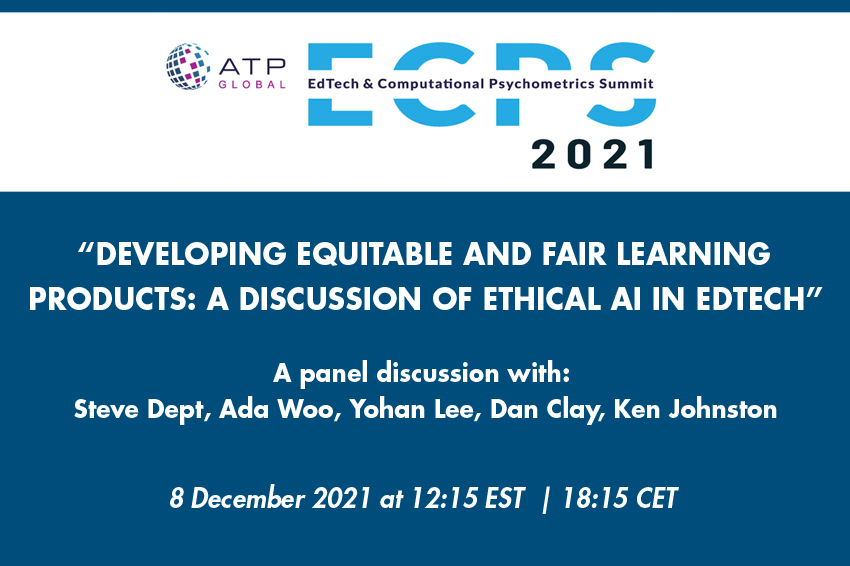
Developing Equitable and Fair Learning Products: A Discussion of Ethical AI in EdTech at the ATP EdTech and Computational Psychometrics Summit
by Pisana Ferrari – cApStAn Ambassador to the Global Village
The 2021 edition of the ATP EdTech and Computational Psychometrics Summit (ECPS) took place virtually on December 8th and 9th, 2021. On December 8, in the course of a session titled “Developing Equitable and Fair Learning Products: A Discussion of Ethical AI in EdTech”, leaders in AI, ethics & education equity, including our own Steve Dept, co-founder and Strategic Partnerships Director at cApStAn, reviewed the current state of AI in education, shared ethical frameworks in EdTech development, and discussed challenges in the field. Ada Woo, Vice President for Innovative Learning Sciences at Ascend Learning, moderated the debate. The other panelists, coming from diverse backgrounds and fields of expertise, were Daniel Clay, Dean, College of Education at the University of Iowa, Ken Johnston, Principal Data Science and Data Engineering Manager at Microsoft, and Yohan Lee, Chief Strategy Officer, Riiid Labs. All had remarkable input, and it was refreshing to hear how ethics is at the heart of the concerns of AI innovators. Ada prompted each panelist to express his views, and the resulting conversation was intense, lively and though-provoking. A recording of the session is due to be released shortly by ATP. Meanwhile, here is a brief summary of the points made by Steve Dept on the issues of data privacy, cultural bias in AI and the role of teachers in EdTech.
Data privacy
One of the issues raised in the discussion was how to ensure that user data are protected and used only for intended purposes, and what Edtech providers can do to foster public trust and data privacy.
Steve replied that in Europe GDPR (General Data Protection Regulation) is now high on the agenda of public institutions and private organisations in EdTech. There is a genuine scrutiny, there are whistleblowers, and the concern that personal data might be identifiable is real. In an international large scale assessment with a data collection with ~5000 respondents per country, it was discovered that a security breach in one country’s system potentially made it possible to trace back responses to individuals, and the data collection was immediately put to a halt in that country.
Cultural bias
AI and algorithms present an air of neutrality but sometimes algorithms perpetuate the dominate culture or dominate language. How can we develop algorithms that advance diversity, equity, and inclusion? And not just maintain the status quo?
Steve said that the output of an algorithm is and remains a statistical prediction. The prediction may be about a learner’s ability to process content; about the best possible translation of text fragment into a given language for a given type of text; or about the match between a test taker’s profile and a job description. Even when data linked to social and emotional skills is added to the mix that generates the prediction, there is nothing more than computation at play. One major risk is that AI will interpret the frequency of certain patterns in the data as factual information. There can be no awareness of a broader reality, there is no intelligence. If this frequency is accidental, or anecdotal, or induced by some sort of bias, the accident or the anecdote or the bias will be replicated in the prediction, and perpetuated. Since AI has become more prominent in education and other high-stakes industries, there is an increased need and demand to trust automated decision-making processes. Only data scientists or engineers – who may not be aware of the biases in the data they use to train the models, and may not be aware of their own biases – understand the backend process and complex deep neural networks. That is why XAI (Explainable AI) has come into being. XAI tools look at the readability of machine learning (ML) models. They help you understand (and interpret) predictions, and allow for non-tech practitioners and stakeholders to be more aware of the modeling process.
Is AI replacing teachers?
One of the goals of using AI in Edtech is to deliver quality education at scale. Is AI replacing the human experiential aspect of learning? Are we trying to replace teachers with AI tools?
Teachers have too much work, said Steve, and much of that work is repetitive and their role is undervalued. What we expect from AI in EdTech is that repetitive tasks can be taken over to free time and energy for teachers to interact with the learners more often and in more meaningful ways, to make their job more rewarding. If there are good results and more coherence in automated coding of essays, for example, it is healthy to invest in that field — and also to use the data to supplement automatically generated feedback with empathy, encouragement, focused support to low achievers, and prompting high achievers to explore a more challenging path.
Session Abstract
A.I., machine learning, and automation are transforming the way we learn. These new technologies hold tremendous promise in democratizing education, by providing learners the tools to gain new skills anytime, anywhere. While A.I. promises to transcend human limitations and increase productivity, it also poses the danger of perpetuating societal biases and amplifying them on a grand scale. How should EdTech providers develop learning products that are ethical and fair? How do we ensure that our A.I. creations reflect human values? In this session, leaders in A.I., ethics, and education equity will review the current state of A.I. in education, share ethical frameworks in EdTech development, and discuss challenges in the field.